Microsoft unveiled Phi-2, a compact or small language model (SLM), in a ground-breaking development in the fields of artificial intelligence and large language models (LLMs). Phi-2 is positioned as an improved version of Phi-1.5 and is available via the Azure AI Studio model catalog at this time.
Microsoft claims that in several generative AI benchmark tests, this new model can outperform bigger competitors like Llama-2, Mistral, and Gemini-2.
After Satya Nadella announced at Ignite 2023, Phi-2 was unveiled earlier this week. It is the outcome of the work done by Microsoft’s research team.
It is claimed that the generative AI model has qualities like “logical reasoning,” “language understanding,” and “common sense.” According to Microsoft, Phi-2 can even do better on some tasks than machines 25 times its size.
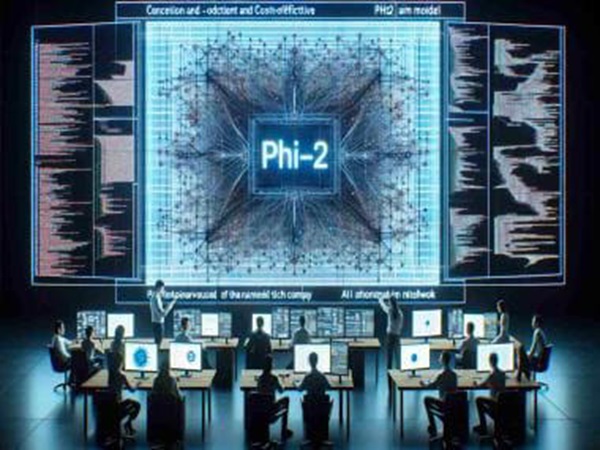
Phi-2 is a transformer-based model that was trained to utilize “textbook-quality” data, which included artificial datasets, general knowledge, theory of mind, everyday activities, and more. One of its features is a next-word prediction target.
Compared to larger models such as GPT-4, which Microsoft claims takes 90-100 days to train utilizing tens of thousands of A100 Tensor Core GPUs, Phi-2 is easier to train and less expensive.
Beyond just processing words, Phi-2 can handle challenging physics and math problems, solve difficult mathematical equations, and spot mistakes in student calculations. In benchmark testing, Phi-2 has fared better than models like the 13B Llama-2 and 7B Mistral in areas including math, coding, language comprehension, and commonsense thinking.
Notably, it runs noticeably better than the 70B Llama-2 LLM and even exceeds the 3.25B Google Gemini Nano 2, which is optimized to run natively on the Google Pixel 8 Pro.
Small language models, which provide a variety of advantages over large language models (LLMs), which are far more widespread, are becoming formidable competitors in the quickly developing field of natural language processing. These models address particular use cases and contextual requirements.
Computational Efficiency: Small language models are more practical for users with fewer resources or on devices with lesser processing capabilities since they require less computational power for both training and inference.
Swift Inference: Smaller models are more suitable for real-time applications where low latency is critical to success since they have faster inference times.
Resource-Friendly: Compact language models are perfect for deployment on devices with limited resources, such as smartphones or edge devices, because they are designed to use less memory.
Energy Efficient: Small models are more energy-efficient during training and inference because of their smaller size and lower complexity, making them suitable for applications where energy efficiency is a key consideration.
Reduced Training Time: Compared to their larger counterparts, training smaller models takes less time, which is a big advantage in situations where quick model iteration and deployment are crucial.
Enhanced Interpretability: It’s usually easier to interpret and comprehend smaller models. This is especially important for applications (e.g., medical or legal) where model interpretability and transparency are critical.
Cost-Effective Solutions: Little models are less expensive to train and implement in terms of time and computer resources. They are a good option for people or organizations on a tight budget because of their accessibility.
Tailored for Specific Domains: A smaller model might work better and be more appropriate than a large, general-purpose language model in some niche or domain-specific applications.
It is important to stress that the choice between large and small language models depends on the particular needs of each activity. Small models are proven beneficial in situations when efficiency, speed, and resource limits are of utmost importance, whereas huge models are highly effective in capturing complex patterns in heterogeneous data.
- Google Keep May Unveil AI-powered “Help Me Create a List” Feature for Shopping, Trips, and More
- Facebook has issued a warning to 1 million users about stolen usernames and passwords
- Unleashing the Metaverse: Microsoft Teams’ 3D Meetings with Mesh
- Could High-flying Drones Power Your Home One Day In Future?
31 comments
[…] Technology […]
[…] Technology […]
I like this site very much, Its a real nice office to read and find information. “Do pleasant things yourself, but unpleasant things through others.” by Baltasar Gracian.
I was examining some of your articles on this internet site and I think this web site is real instructive! Keep on putting up.
There are some fascinating cut-off dates in this article but I don’t know if I see all of them center to heart. There may be some validity but I will take hold opinion till I look into it further. Good article , thanks and we wish extra! Added to FeedBurner as properly
I like this weblog so much, bookmarked. “I don’t care what is written about me so long as it isn’t true.” by Dorothy Parker.
you are really a good webmaster. The web site loading speed is incredible. It seems that you are doing any unique trick. Furthermore, The contents are masterpiece. you’ve done a great job on this topic!
Hi, i think that i saw you visited my weblog thus i came to “return the favor”.I’m trying to find things to enhance my site!I suppose its ok to use some of your ideas!!
You have noted very interesting details ! ps decent website .
Hi just wanted to give you a quick heads up and let you know a few of the pictures aren’t loading properly. I’m not sure why but I think its a linking issue. I’ve tried it in two different browsers and both show the same outcome.
You made a few fine points there. I did a search on the topic and found a good number of folks will agree with your blog.
Wow! Thank you! I constantly wanted to write on my blog something like that. Can I take a fragment of your post to my blog?
I am impressed with this site, real I am a big fan .
I like what you guys are up also. Such clever work and reporting! Keep up the excellent works guys I’ve incorporated you guys to my blogroll. I think it’ll improve the value of my web site :).
It is best to take part in a contest for among the finest blogs on the web. I will suggest this website!
Utterly written content material, thankyou for selective information.
I used to be very happy to find this internet-site.I wanted to thanks for your time for this excellent read!! I undoubtedly enjoying each little little bit of it and I’ve you bookmarked to take a look at new stuff you blog post.
Only a smiling visitant here to share the love (:, btw great design and style.
I like what you guys are up also. Such smart work and reporting! Keep up the superb works guys I?¦ve incorporated you guys to my blogroll. I think it’ll improve the value of my site 🙂
Please let me know if you’re looking for a writer for your site. You have some really great articles and I think I would be a good asset. If you ever want to take some of the load off, I’d really like to write some content for your blog in exchange for a link back to mine. Please shoot me an email if interested. Cheers!
Thanks for the sensible critique. Me and my neighbor were just preparing to do a little research about this. We got a grab a book from our area library but I think I learned more clear from this post. I am very glad to see such magnificent info being shared freely out there.
What is Sugar Defender 24? Jeffrey Mitchell made the Sugar Defender 24. It is a product (Sugar Defender Diabetes) that helps your blood sugar health.
I like this web site so much, saved to favorites.
Simply a smiling visitor here to share the love (:, btw outstanding layout. “He profits most who serves best.” by Arthur F. Sheldon.
Some genuinely good info , Sword lily I found this. “What’s a man’s age He must hurry more, that’s all Cram in a day, what his youth took a year to hold.” by Robert Browning.
I really like your writing style, great information, appreciate it for posting : D.
I am perpetually thought about this, thanks for putting up.
This really answered my problem, thank you!
This internet site is my aspiration, really superb style and perfect subject material.
I will immediately grab your rss feed as I can not find your e-mail subscription link or newsletter service. Do you’ve any? Kindly let me know so that I could subscribe. Thanks.
Hi there, i read your blog occasionally and i own a similar one and i was just curious if you get a lot of spam comments? If so how do you reduce it, any plugin or anything you can suggest? I get so much lately it’s driving me crazy so any support is very much appreciated.